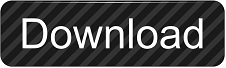

Full factorial analysis of the, resulting microarray data revealed that collagen IV exhibited the highest positive effect on cell attachment This data processing approach allows for fast and unbiased analysis of cell-based microarray data. or juxtaposed cells that identify positive for both markers due to close spatial. We couldnt find any CENP-F positive cells over 266 points of cell nuclei. Combined, the approach identified 78% of high quality spots and 87% of poor quality spots. microscopy processing tilescans in CellProfiler Getis-Ord spatial. Open source programs, such as FociCounter and CellProfiler, are used to. Naive Bayesian classifiers trained on manually scored training sets identified good and poor quality spots using spot size, number of Cells per spot, and cell location as quality control criteria.
#Cellprofiler identify postive cells software#
Most times the manufacturer of your microscope has some software for 3D visualization / rendering, or.
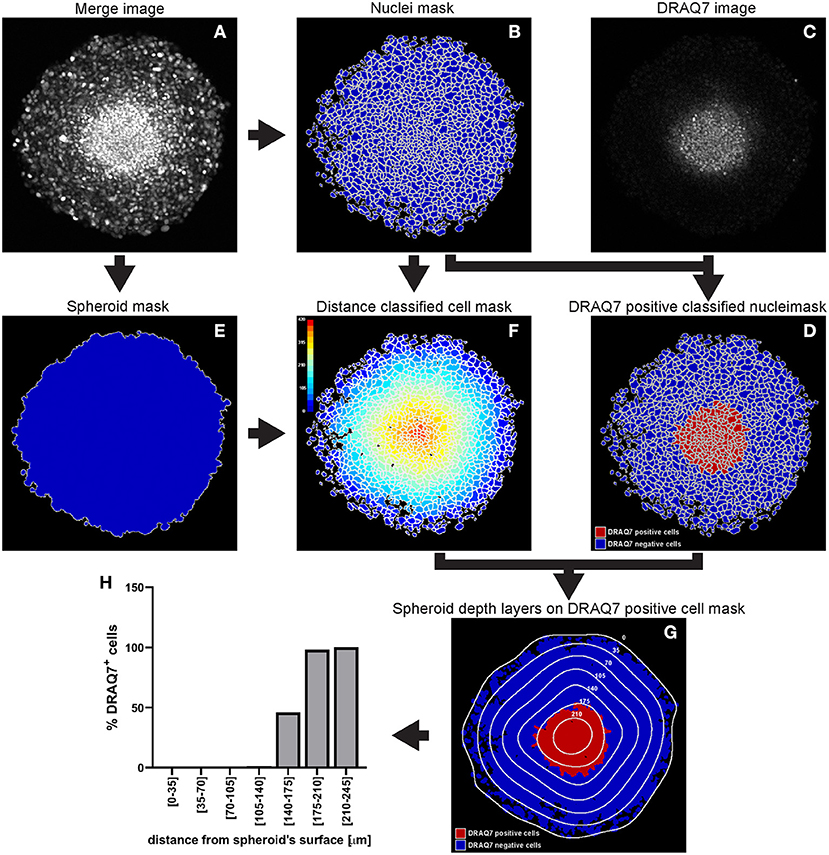
InputImages -plugins-directory. At the moment, CellProfiler can only analyse 2D images or individual images in a stack. From these images, clusters of cells making up single cell spots were reliably identified by analyzing the distances between cells using a density-based clustering algorithm (OPTICS). For either pipeline, either open CP, load the CHARM-like pipeline you want and run analysis, or run CP headless (from CellProfiler directory) using the following command: python CellProfiler.py -p. Microarrays were imaged by automated fluorescence microscopy and cells were identified using open source image analysis software (CellProfiler). The approach was used to analyze the adhesion of murine cardiac side population cells on combinatorial arrays of extracellular matrix proteins. Alternatives: Image analysis softwares capable of identifying fluorescent positive cells such as ImageJ ( Schneider et al., 2012) or CellProfiler may be used to assess TO viability from images generated during the experiment. This work introduces an automated approach to identify cell based microarray spots and spot quality control. Optimize fluorescence intensity thresholds to identify fluorescent positive cells for each reagent individually. Previously, microarrayed cell spot identification and quality control were performed manually, leading to excessive processing time and potentially resulting in human bias. While high content image analysis, cell counting, and cell pattern recognition methods are established, there is a need for new postprocessing and quality control methods for cell based microarrays used to investigate combinatorial microenvironments. Analysis of microarrays requires several steps, including microarray imaging, identification of cell spots, quality control, and data exploration. (B) Viability values from positive and negative control well replicates are used to assess assay quality by Z-factor (left) and coefficient of variance.
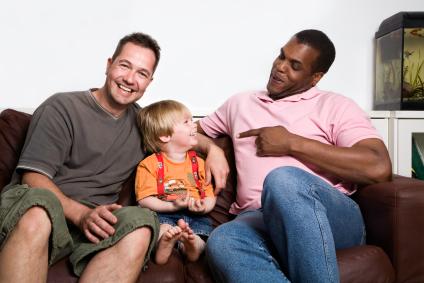
Cell-based microarrays are being increasingly used as a tool for combinatorial and high throughput screening of cellular microenvironments. Hoechst is used to identify the nuclei of all cells, Caspase-3/7 activated reagent is used to identify apoptotic cells, and TO-PRO-3 is used to identify all dead cells.
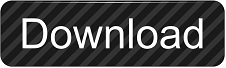